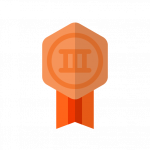
EPIC-KITCHENS [1] is the largest-scale egocentric dataset collected by 32 participants in their native kitchen environments, and densely annotated with actions and object interactions (125 verb classes and 331 noun classes).
The dataset is aligned with six challenges: action recognition (full and weak supervision), action detection, action anticipation, cross-modal retrieval (from captions), as well as unsupervised domain adaptation for action recognition.
The unsupervised domain adaptation challenge tests how models can cope with similar data collected 2 years later on the task of action recognition. The goal is thus to assign a (verb, noun) label to a trimmed segment, following the Unsupervised Domain Adaptation paradigm: a labelled source domain is used for training, and the model needs to adapt to an unlabelled target domain. Videos recorded in 2018 (EPIC-KITCHENS-55) constitute the source domain, while videos recorded two years later (EPIC-KITCHENS-100’s extension) constitute the unlabelled target domain.
Our PhD students Mirco Planamente and Chiara Plizzari achieved the 3rd place position [3] in the third edition of the challenge, presented at the Eighth International Workshop on Egocentric Perception Interaction and Computing. They re-purposed the Relative Norm Alignment loss [2], a multi-modal loss recently proposed to deal with the Domain Generalization setting for action recognition, to operate between different backbone architectures in order to enhance their collaboration. Indeed, they achieved top performance on all verb, noun and action category.
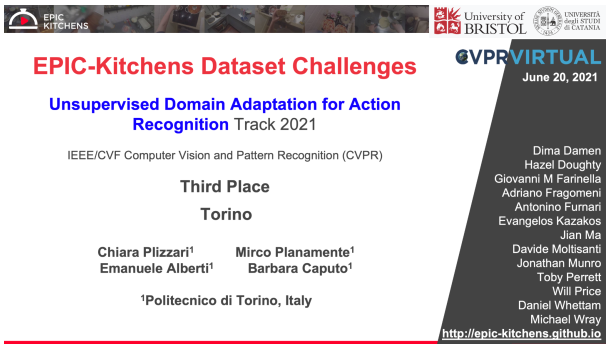
[1] Damen, Dima, Hazel Doughty, Giovanni Maria Farinella, Sanja Fidler, Antonino Furnari, Evangelos Kazakos, Davide Moltisanti et al. “Scaling egocentric vision: The epic-kitchens dataset.” In Proceedings of the European Conference on Computer Vision (ECCV), pp. 720-736. 2018.
[2] Planamente, Mirco, Chiara Plizzari, Emanuele Alberti, and Barbara Caputo. “Domain Generalization through Audio-Visual Relative Norm Alignment in First Person Action Recognition”, WACV 2021.
[3] Plizzari, Chiara, Mirco Planamente, Emanuele Alberti, and Barbara Caputo. “PoliTO-IIT Submission to the EPIC-KITCHENS-100 Unsupervised Domain Adaptation Challenge for Action Recognition.” arXiv preprint arXiv:2107.00337 (2021).
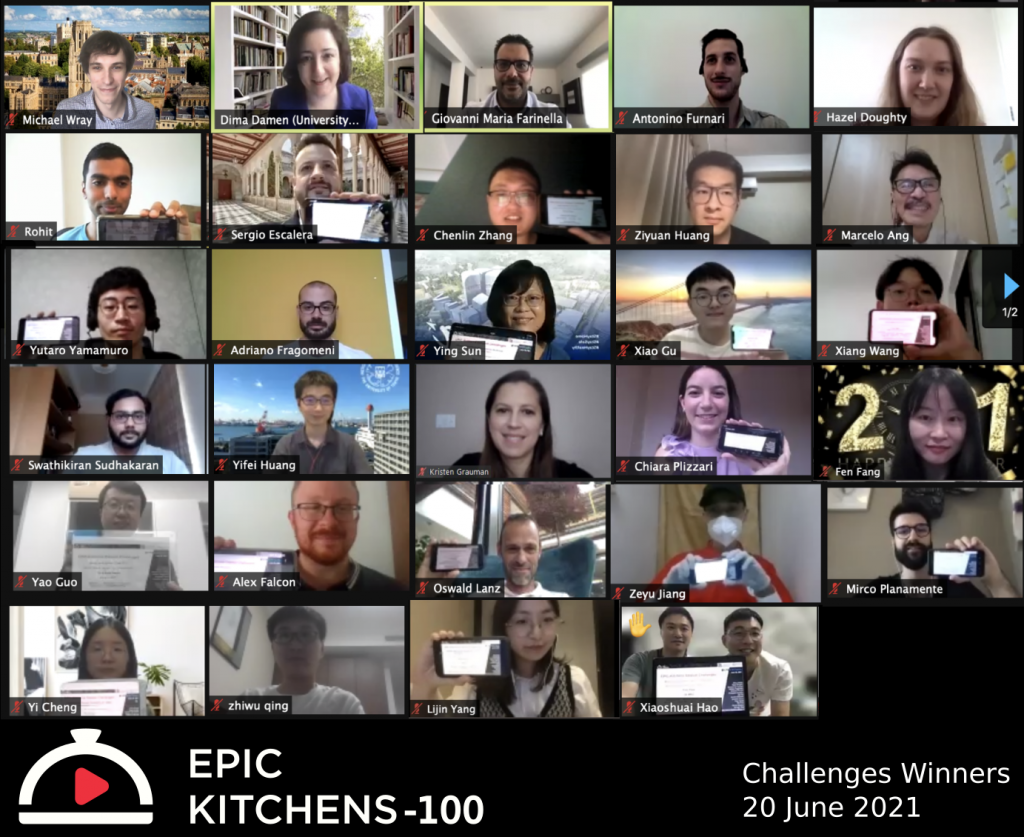